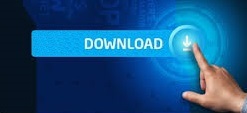

Deploy the solution rapidly, without any disruption.Is it easy to integrate? Could you get started today? How many sprints would it take to fully integrate with your platform?Īt SEON, we put flexibility and customization at the core of all our anti-fraud features.Is it expensive? Will you pay per API call or as a lump sum for a multi-year contract? Micro-fee vs chargeback guarantee?.Is it scalable? How many rules does your system support? Can you run thousands of API calls per day? And, if it’s hosted online, what kind of uptime rate are you looking at?.An iGaming company has very different needs from, say, a neobank. And more importantly, consider whether you can deploy the right rules based on your vertical. Finding a solution that lets you stack rules, use fraud scoring, custom fields, or even machine learning suggestions will reap better rewards in the long run. Is it flexible? While rule-based systems sound inflexible, it doesn’t have to be the case.Is it effective? Are you getting the results you were hoping for? Some fraud providers favor an approach based on shared blacklists, while others prefer relying on the power of real-time data.Still, some key fundamentals should apply to your rule-based fraud detection solution: There are as many fraud prevention strategies as there are businesses.
#Manual rules risk engine how to#
How to Choose a Rule-Based Fraud Detection Solution Thanks to machine learning, you can even get suggestions that are relevant based on your unique business use case. This means you can monitor results, set KPIs, and tweak rules to align with your strategy.

Rule-based fraud prevention is data-driven. To ensure you don’t lose any good customers, you can introduce extra checks for those who require a little extra vetting instead of blocking them outright. That means obvious fraudsters are blocked and legitimate users are let through with no friction. While it’s possible for a small business to manually review each user action, you can only really scale your fraud prevention strategy by automating checks based on fraud rules.Ī great benefit of rule-based detection is that you can still manually review cases that fall within a grey area. However, there are undeniable benefits, including: Benefits of a Rules-Based Fraud Prevention SolutionĪs you can see from the examples above, rule-based fraud prevention varies greatly depending on the kind of rules you deploy. On the SEON platform, the machine learning engine runs in real-time and gathers data from transactions and scoring as it happens.Īs a result, the more you use it, the more accurate the suggestions are going to be – while their remain easily explainable thanks to the whitebox nature of the module. You’ll need to manually review and test them yourself – either on historic data or on live data in a sandbox environment. Of course, AI (or machine learning in our case) won’t know if the rules are good or not. The first parameter will flag credit card details that point to the US. Your static rule could be made up of two parameters. Let’s say, for instance, that you have access to IP information and credit card details. However, static rules can be as creative as you want based on the data you have at hand. If an IP was found on a blacklist, the user would be blocked. Historically, the earliest static rules had to do with IP addresses. It is considered static when the outcome of the rule is strict and inflexible – for instance, blocking a user action. Static Rules for Fraud DetectionĪ static rule is the most basic form of fraud rule and tends to follow a simple if/then logic. Let’s look at five different types of rules with clear examples based on the SEON platform. SEON is a powerful end-to-end solution that gives you complete control over the rules that affect your users’ fraud scoresīook a Demo Rule-Based Systems for Fraud Detection
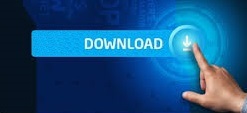